Understanding AI in Clinical Trials: Where Do We Begin?
- Tryal
- Apr 10
- 3 min read
Clinical trial study teams recognize that Artificial Intelligence (AI) is becoming an essential tool in the industry. Many feel the pressure to adopt AI and develop an AI strategy but struggle with where to start. The challenge isn’t just about implementation—it’s about understanding what AI is, what it can do, and its limitations.
Too often, AI is presented as a futuristic, catch-all solution. While teams read about AI breakthroughs in the news and see new products integrating AI, they lack the vocabulary and foundational knowledge to assess AI’s real-world applications in clinical trials.
To bridge this gap, here are three core AI concepts that every clinical trial study team should understand.
1. Large Language Models (LLMs)
A Large Language Model (LLM) is an AI algorithm trained to process and generate human language. It works by analyzing vast amounts of text from the internet and predicting the most likely sequence of words based on a given prompt.
Key Limitations:
LLMs don’t make judgments or analyze data like a human would. They predict responses based on patterns rather than critical thinking.
Their knowledge is limited to what they’ve been trained on. If AI systems rely too heavily on sources like social media, the generated responses can be biased or unreliable.
It's not inherently ethical - LLMs can have a hard time understanding certain social norms, so some clinical initiatives like study diversity or recruitment could be problematic if depending on AI to recognize all relevant social norms or limitations. For clinical trial teams, this means that while LLMs can assist in drafting reports or summarizing information, they should not be relied upon for critical decision-making without human verification.
2. AI Hallucinations
Hallucination is a common AI phenomenon where an AI model generates false or misleading information because it is designed to always provide an answer, even when it lacks the necessary data.
Why It Matters in Clinical Trials:
AI-generated hallucinations can be harmless in creative fields, but they pose significant risks in clinical research. If AI is used to summarize clinical data or answer regulatory questions, its output could contain inaccuracies that may not be immediately obvious. The greatest risk is that if a user does not already know the answer, they may not realize when AI is providing incorrect information.
To mitigate this risk, clinical teams need verification processes and full output traceability in place to ensure AI-generated content is relevant and accurate before it is used in critical applications.
3. Safe Application of AI in Clinical Settings
Applying AI safely in clinical trials means ensuring transparency, accountability, and regulatory compliance.
Best Practices:
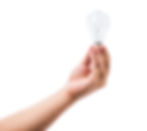
Transparency: AI-generated outputs should always include references and traceable sources, similar to how an intern would be expected to show their work.
Audit Logs: AI-driven processes should be accompanied by documentation and validation to ensure accuracy.
Checks and Balances: Secondary review systems should verify AI-generated content before it is applied to clinical decisions.
At Tryal, we take a structured approach to AI adoption, ensuring that all AI-driven insights are transparent, reviewable, and compliant with industry regulations.
Common Misconceptions and Risks of AI in Clinical Trials
Many professionals fall into two extremes:
Blind Adoption – Rapid AI adoption without fully understanding its limitations can lead to serious risks.
Complete Avoidance – Fear of AI can result in missed opportunities for efficiency and innovation.
A prime example is the over reliance on ChatGPT for clinical content. Asking AI a clinical question and pasting the response into a controlled system is akin to taking advice from a random person without verification. When put in these terms, it’s clear why unchecked AI adoption can be risky.
Building a Safe, Educated Future for AI in Clinical Trials
AI is not something to fear—but it does require education and careful implementation. At Tryal, we are committed to helping clinical teams navigate AI adoption safely. By prioritizing education, transparency, and compliance, we ensure that AI enhances clinical research without compromising quality or safety.
Understanding AI is the first step toward leveraging it effectively in clinical trials. With the right knowledge and safeguards, study teams can confidently integrate AI into their workflows to improve efficiency and decision-making while maintaining regulatory compliance.